Personalized Content Recommendations: How AI and Machine Learning are Revolutionizing Apps
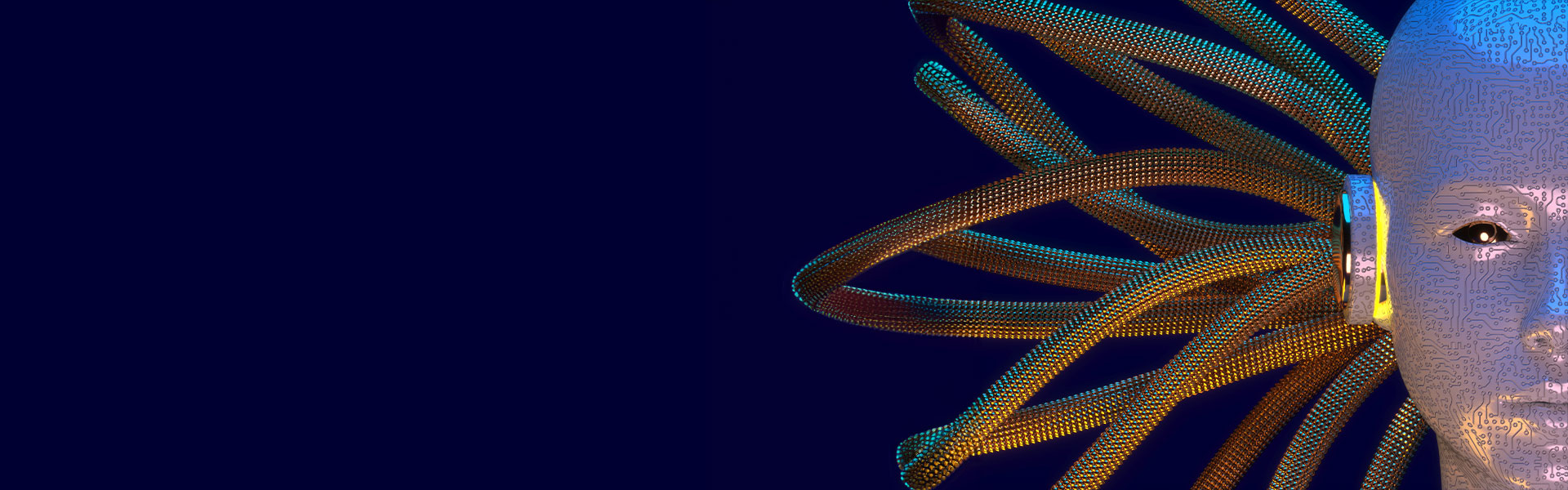
As apps and online services compete for our time and attention, many turn to artificial intelligence (AI) to deliver a more personalized experience. With intelligent algorithms, apps can learn our preferences and interests and customize content and product recommendations tailored just for us.
For users, AI-powered personalization means seeing the stuff we want to see. It translates to higher engagement, sales, and customer loyalty for businesses.
Understanding Users through Data Collection
The critical ingredient for personalized recommendations is data – and lots of it. Here are some ways apps gather intelligence on their users:
Tracking Content Interactions: By monitoring the content we view, like, share, or purchase, apps pick up clues about our interests to refine recommendations. We’ll likely see more baking content if we save banana bread recipes.
Building Detailed Profiles: Registration forms request details like age, location, and gender to segment audiences. Filling out our profiles helps apps discern demographic-based interests.
Evaluating Metadata: Products have descriptions, categories, and price points. Analyzing this metadata enables apps to find related items to suggest.
Considering External Factors: Our usage depends on the context, like weather, holidays, and current events. If it’s raining out, fitness apps may push indoor workouts. Around the holidays, e-commerce apps promote gift ideas.
Applying Machine Learning Models
Machine learning models can uncover hidden insights and patterns with rich user and product data collected. Models are trained to analyze the various data signals to determine each user's interests, preferences and behavior. Common machine learning techniques include:
Collaborative filtering - Analyzing patterns across similar users to find correlations and make predictions. Users who interacted with A also interacted with B.
Content-based filtering - Recommends identical items based on their attributes, keywords, metadata, etc. You viewed product X; here are similar products.
Contextual modeling - Considers real-world context like time, weather, and location to recommend relevant timely content. It's Saturday morning, here are brunch spots nearby.
Natural language processing - Understands text-based interactions to infer sentiment, and topics of interest, and detect user intent. User searches for "outdoor birthday venues", recommends relevant options.
Neural networks & deep learning - Advanced techniques that uncover latent features and patterns through multilayered processing. Great for highly personalized recommendations.
Delivering Personalized Experiences
Once user interests and preferences are determined, apps can provide tailored experiences through:
Customized content feeds - For your pages with articles, products, and posts filtered to each user's needs.
Targeted ads - Promotions and sponsored content match user interests for higher engagement.
Product recommendations - Suggested items based on purchase history, browsing, wishlists and more.
Featured or suggested content - Spotlighting new releases, and topics and more likely to appeal specifically to the user.
Email newsletters - With curated products and content personalized for subscribers based on past interactions.
Adaptive interfaces - Changing UI elements, information hierarchy and content displayed based on the individual.
Benefits of Personalization
Effective personalized recommendations can provide multiple benefits:
Increased user engagement - More time spent exploring relevant content and customized products.
Higher conversion rates - More clicks, purchases and signups from personalized recommendations matching user intent.
Improved customer experience - Users feel understood and satisfied with tailored recommendations. Builds loyalty.
Reduced churn - Users remain engaged rather than leaving due to irrelevant generic content.
Competitive differentiation - Personalization sets apps apart and promotes user retention in a crowded market.
Deeper insights - Analyzing user behavior provides valuable data to refine recommendations and business strategy.
Considerations for Implementation
Here are some tips when implementing a personalized recommendation system:
Prioritize privacy and obtain proper user consent. Transparency builds trust.
Focus recommendations on value, not maximizing engagement. Avoid intrusive ads.
Balance personalization with diversity. Don't limit users to echo chambers.
Evolve algorithms continuously based on feedback and testing. Preferences change.
Consider context and timing. Recommend birthday gifts before their birthday, not after.
Explain why recommendations are shown. Users want to understand relevancy.
With the power of AI and machine learning, apps can tap into personalized content recommendations to tailor experiences uniquely to each customer. When done right, the results are happy users, improved engagement metrics, and tangible business gains. The future lies in intelligent systems that know users better than they know themselves.
Powered by Froala Editor